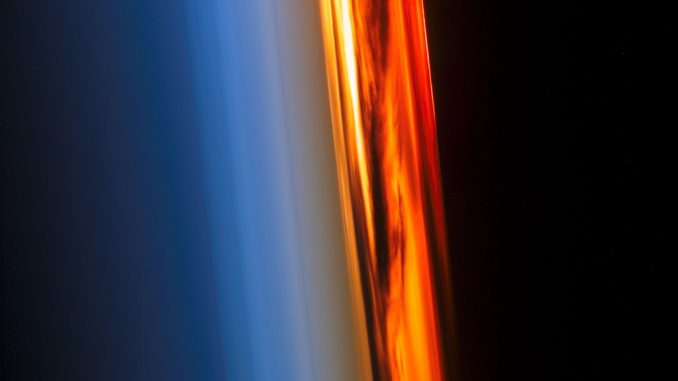
Time to thoroughly tackle a tough topic: trust and traceability in science. I’m sure we won’t tackle it for science in general, but at least we can think about it in the framework of the workflows in our lab…
Generally speaking, scientific findings are conclusions, drawn from an interrelated amalgamation of information. A reasonable effort is done by the scientist when writing a paper to document this amalgamation, but a lot of information is omitted from these publications, either by convention, for brevity, or for space reasons.
If we want to get people to trust these scientific findings more, we need to make the entire argumentation path traceable. Once that is done, a level of trust can be assigned independent of the scientist who did the work (in an ideal world at least). Bonus points if your work is so traceable that it can be reproduced.
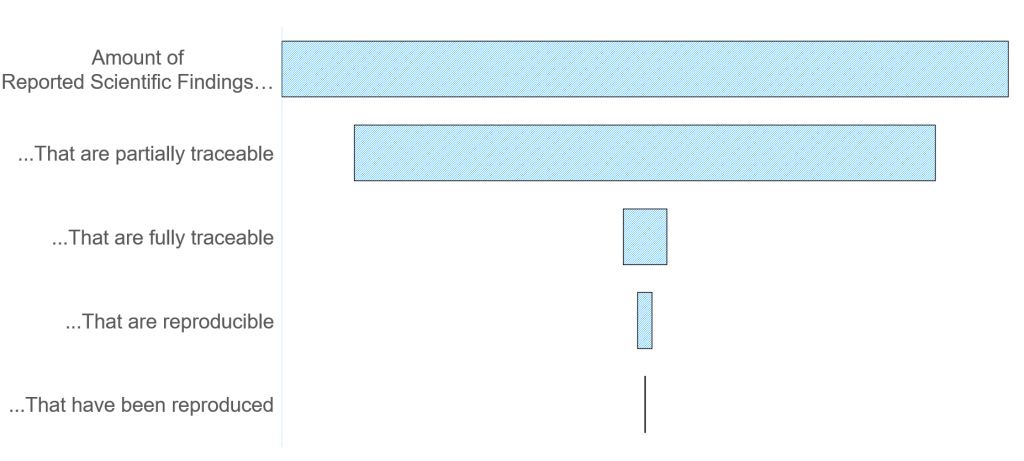
There have been plenty of efforts to make science more traceable and reproducible, but many of these are limited to a technique, a dataset, or an analysis. As a gedankenexperiment*, let’s see what would be needed to traceably document a complete experiment in our MOUSE lab.
In my presentations I have always presented the following simplified workflow, so we can use that as a starting point. We need to cover aspects of sample preparation and sample selection, the measurement process, the data correction aspects, the (multiple) analysis details and how the analyses are turned into an interpretation (or a finding):

That, of course, is overly simplified. When we work this out a bit, we would be able to expand this to the following workflow (click to enlarge):

This shows the individual steps and the quantities of files we are dealing with for a batch of measurements. Fortunately, a significant chunk of this can be automatically processed automatically with our RunDeck instance:

For traceability, we are storing several pieces of information in our SciCat measurement catalog, including information on the user, the samples and the project:

This already looks quite promising, but once you take another step back, you will see that we are still not capturing a lot of scientific (meta-)data and domain knowledge essential to arriving at the conclusion. Examples are information on codebases (repository links and commit IDs would suffice here), but also sample history, project scope and timeline, configuration files, masks, other analyses, domain knowledge and literature links:
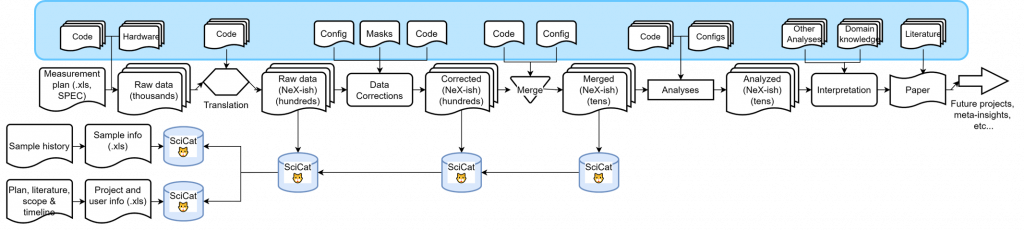
In an ideal world, we would catalog many of these auxilary files and links, and link them to the workflow that was used to arrive at particular scientific findings. This only requires time, and an in-depth understanding of the scientists of the workflow and information they used to get somewhere. We will be spending some time to try to capture more of this into scicat, and see how far we can get.
*) and, in fact, for a presentation I gave for the SciCat user meeting this week…