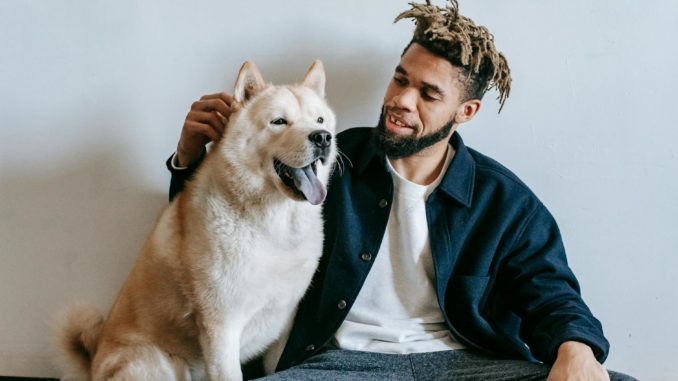
“How can I find out what the good papers in a field are”, asked a student at my last Dark Side of Science talk. That is a good question worth delving into, and there may be a partial solution to that: the idea of Peer-Assigned Trust (signatures), or PATs for short.
What’s the problem with trust in science?
The metrics-based incentives imposed upon us have led us to the point where we now face an avalanche of new papers whose trust and reliability is far from guaranteed. This has led to fundamental and growing problems in science related to trustworthiness, and started discussions on replacing academic journals altogether. Can this be solved with more stringent peer reviews, AI-assigned “quality” scores or automated reviews? As Betteridge’s law tells us: probably not. These solutions are trying to fight the symptoms, and not the root of the problem.
The anonymous peer review system that became the norm in the 1960s, was supposed to create more trust in science. Until then, journals mainly published works their staff thought worthwhile. While peer review has slowed down science significantly to the point where it is not uncommon for a paper to spend a year in peer review, it has demonstrably failed to stop bad science, and in its current form can not be considered an adequate measure to ensure a standard of quality in published papers.
Publishers are now looking towards AI to alleviate their woes, which, crudely speaking, is a glorified autocorrect tool without reasoning capabilites. AI has its place, I use it a lot to speed up coding, for example, but assessing scientific papers is not one of them. Nevertheless, there’s a lot of AI-woo being peddled for this purpose at the moment, purported uses include assigning “quality” scores to manuscripts, assigning novelty metrics, or even automating peer review (same article). And even if all of this would work well, it is still unlikely to improve the science publishing landscape as we are not addressing the incentives that lead to this torrent of low-quality papers in the first place.
Addressing the root of that problem would look different, something along the lines of only allowing a researcher to have their name on a maximum of two papers per year. Now you have created a driving force to making these papers impactful, and focusing a scientist’s effort on doing a few projects well, rather than as many as possible (this could be combined with innovations in the grant landscape to reduce waste of academic time). However, I cannot see such a drastic change happening in my lifetime.
So what is an busy scientist to do? How do you find out what papers to trust and which ones are worthwhile?
A possible solution: build a network of trusted peers
One solution can be found in other places such as sailing, book and movie reviews, social media…
As a sailor, for example, you are offered a multitude of products that are supposed to make your life easier and safer. But many of these are garbage, so you rely on the recommendations of a handful of other experienced sailors – people you trust. We can apply the same to papers, datasets and other scientific work; we can assign a signed marker of trust to pieces of work (or even people), akin to an your friends “like”-ing a post on social media, or a trusted book review on Goodreads…
So if a paper or dataset is trusted by several of the people that I trust, that paper is probably quite trustworthy. More precisely, we should probably consider (five-star-)rating the most important aspects of a paper or dataset separately:
- The methods / experimental section: Although in some journals the experimental section is relegated to a less prominent spot, the methods form the foundation on which the paper is constructed. Are the methods trustworthy, described with care and with sufficient detail to make it possible to reproduce? Are the processing and analysis equations and associated assumptions appropriate, did they use all or most relevant characterisation methods? tl;dr: do you trust the data these people obtained?
- The results and discussion: Do you trust the results, the fits, and the interpretation the authors eked out of these? How did they deal with the inconsistencies between the results from the different methods? Do they discuss the limits, outliers, uncertainties?
- the conclusions: Do you believe the authors could draw those conclusions from the data and analysis, or did they overreach, oversell, overpromise?
With this differentiation, you can say “I trust this dataset to be good and the methods to be sound, but the analysis is a pile of rubbish”. These trust markers could be digitally signed along the lines of the PGP/GPG cryptographic signatures, i.e. those that do not require a central agency doling out keys (remember key-signing parties?). There’s also no reason to limit this to preprints, papers and datasets, you could publicly affirm your trust in certain people as well.
The good thing about such an approach is that you can decide what your network of trust looks like, and how many degrees you want to trust beyond. For example, if I trust person A to know what they are talking about when it comes to scattering, I might (perhaps to a lesser degree) trust the people they trust. I could also say I trust this person when it comes to scattering, but not on other topics.
You could even trust entire organisations if you want, though perhaps as a different class of trust as you are essentially blanket-trusting a diverse smattering of people. Of course, some degree of privacy is required in both cases to prevent revealing that you maybe don’t trust your colleague’s work all that much. For the brave, perhaps we can also have negative trust markers, as in “this paper I don’t trust”. I certainly have a few examples of these too, and they are far more illustrative than the good papers.
The main advantage of this is that you would be notified of good papers or good preprints (via ArXiv or Open Research Europe) far quicker through your trust network. Likewise, students can find out which articles are highly recommended in a field after adding a few key people to their (field-specific) network.
But, in a good way, this is not too radical a change from how we currently work. At the moment, we trust certain journals to give us papers with a level of quality and trustworthiness, but in the end that comes down to trusting the people behind it such as the editors and (anonymous) reviewers. With PATs, we short-circuit this process by trusting certain people directly, and augment this network with their trusted peers. In a way, we already see it in action on social media, with people highlighting papers and work of interest besides their own.
So what’s the catch?
There are downsides to this idea too. Like all systems, this can emphasise popular papers, and papers from popular authors. As before, young and upcoming scientists will have to advertise their work at conferences using presentations and posters, and through collaboration with established academicians. This, as before, does not alleviate the risk of clique formation.
PATs, however, would be a lot more transparent by design than the obscure, metrics-driven approach that drives the publication selection process in journals. And open systems are a lot more trustworthy and pleasant to work with. It doesn’t need to be perfect, just better than the current situation.
Now this idea needs a lot of work if it is ever to be implemented, not to mention a sustainable source of funding etc. etc., but as a thought experiment it is a fun tool to play with. In the extreme imaginary (utopian) scenarios, one could do away with journals and classical peer review altogether, whilst increasing quality over quantity, thus bringing us a little bit closer to the essence of science. And just maybe, it will help reduce the stream of bad papers that slowly poisons our entire existence.
One can always hope… Happy new year everyone!