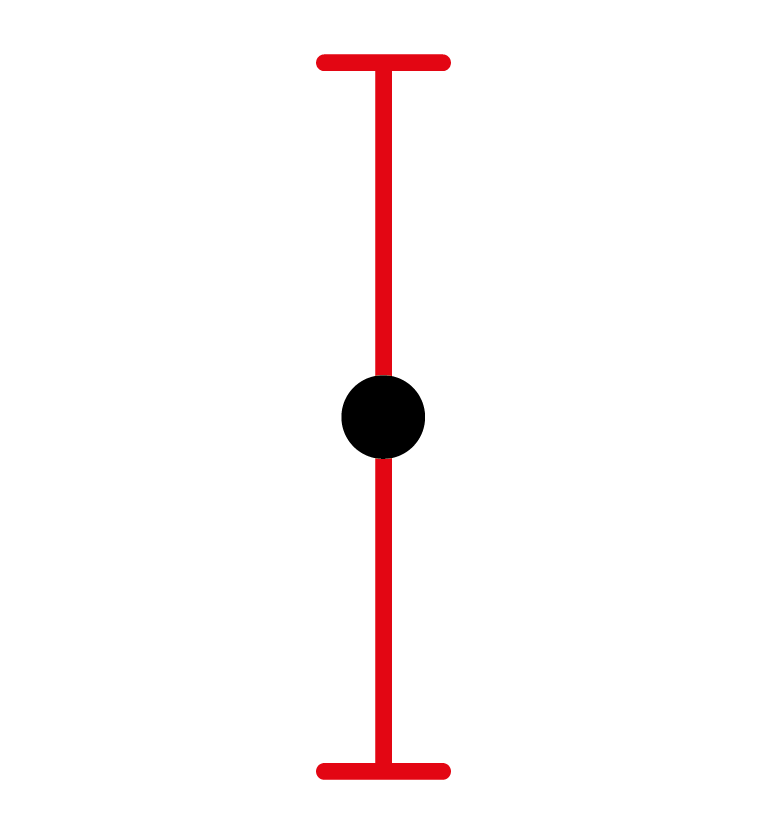
I feel I need to make a public apology: it turns out that I have been inaccurate in some of my recent papers on the explanation of the estimation of the uncertainties on the intensities (the uncertainties themselves, however, are correct, so I calculated it right but described it wrong). Here’s what happened:
Over the years, I have build a bit of a library of programs to process data. One of the oldest pieces from my Ph.D. time was an integration method for integration of 2D scattering patterns, which has since been reprogrammed in Python. The integration method uses two internal uncertainties, one which propagates the uncertainties of the detected counts, and the other determining the standard deviation of the intensities in the bin. Whichever was largest was my estimate for the uncertainty (unless both are less than 1% of the intensity, in which case the error is estimated as that). However, there was an oddity in that calculation: for a reason I forgot, I further divided the standard deviation of the intensities in the bin by the square root of the number of values in that bin. This procedure has remained unchanged since I initially wrote it, and has produced sound results since. However, it is at odds with what I have been writing, my publications state that (one of) the uncertainty estimates is the standard deviation!
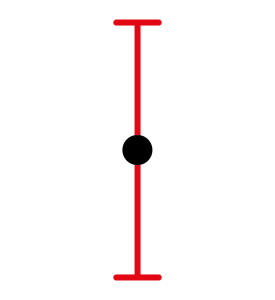
The calculation turns out to be the correct one. The standard deviation of the intensities in a bin will assume a fixed value that indicates how much the binned intensities vary from the mean. For an infinite number of intensities in a bin the standard deviation will therefore assume a finite value. When I want to calculate the uncertainty of the mean determined through the binning procedure, however, I need to calculate the “standard error of the mean“. This value (as you guessed), is the standard deviation divided by the square root of the number of measurement points, and indicates how accurate the mean bin value is. For an infinite number of intensities in a bin, this value will be zero (i.e. the mean is perfectly accurate).
When I was writing the “Improvements and considerations”-paper [1], I got it half right by stating that the uncertainty is the maximum of either the propagated uncertainties, or the “standard error of the mean”. However, when I then wrote the equation for the uncertainty (equation 9), I stated the equation for determining the standard deviation. Worse still, when writing the “Everything SAXS”-paper [2], I stated that it is the standard deviation, and gave the equation for the standard deviation as well. It is furthermore incorrectly stated in [3]. Egg and my face are in alignment (paraphrasing Moss from “The IT Crowd”).
I will do my best to clarify this difference in future work, and maybe should publish a corrigendum to the Everything SAXS paper. I sincerely apologise for any confusion it may have caused to any of you. Feel free to ask me to buy you a compensatory beverage at the next conference.
[1] B. R. Pauw, J. S. Pedersen, S. Tardif, M. Takata, and B. B. Iversen. Improvements and considerations for size distribution retrieval from small-angle scattering data by Monte Carlo methods. J. Appl. Cryst., 46:365–371, 2013.
[2] B. R. Pauw. Everything saxs: small-angle scattering pattern collection and correction. J. Phys.: Condens. Matter, 25:383201, 2013.
[3] J. M. Rosalie and B. R. Pauw. Form-free size distributions from complementary stereological tem/saxs on precipitates in a mg–zn alloy. Acta Materialia, 66:150–162, 2014. arXiv:1210.5366.
Leave a Reply