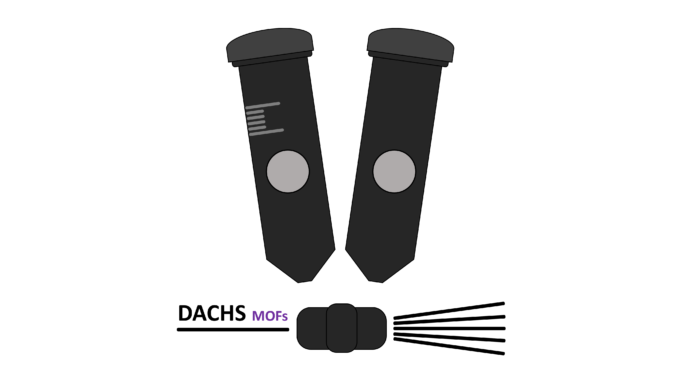
Our RoWaN automated synthesis platform has been used to perform over 1200 ZIF-8 syntheses, each with slight variations in the experimental procedure. We went to extraordinary lengths to comprehensively document the syntheses themselves, including documenting all details of the equipment, chemicals and the step-by-step synthesis procedure. The fine-tuning of this documentation in human- and machine-readable files is now sufficiently advanced that we can call it a version 1.0. To help exploit the wealth of information contained in these libraries, for example through statistical data mining or synthesis replication, we are making the syntheses libraries available per synthesis batch. The first five of these batches, describing 147 syntheses in total, are now available online. Read on to find out more.
The first five synthesis libraries are available on Zenodo, here, here, here, here and here. The first few are small batches made with a very simple set-up (just a syringe injector over a stirring plate), but the later batches are made using the full automated system with the robot arm to control the deposition.
Making these syntheses available serves two purposes: on the one hand, we want to prove (in the classical sense) that the syntheses can be sufficiently well reproduced with the recorded information by independent laboratories, using the information contained in the files themselves. On the other hand, these libraries can serve as a FAIR+trustworthy basis for data mining and machine learning systems, who depend on the availability of this data.
More precisely, since what we call AI is a statistical probability matrix based on the training dataset (and therefore inherently derivative in nature), the quality of the output depends strongly on the quality of the input. If, for example, the AI model is trained with syntheses as reported in literature, essential information such as the stirrer bar shape, chemical addition order and addition rate are likely not present in the training dataset. Our results, however, have shown that these are important factors needed to reproduce a particular ZIF-8 particle morphology. This lack of information in the training datasets means that any AI-generated synthesis procedure will lack critical information needed to get a reliable outcome.
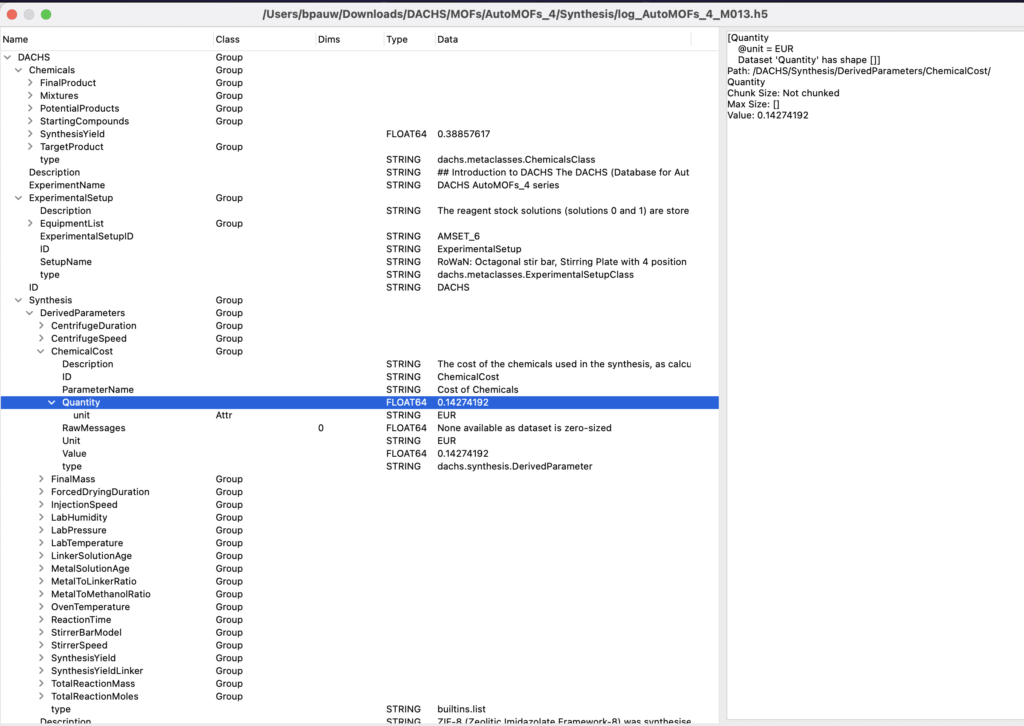
For us, it has also been an important exercise in finding out how much information can be recorded, and what the best way to record this information is. The structure of the HDF5 files containing the synthesis details has been adjusted, so that the source and derived information is present, and that it can be read by machines but also understood by humans. There are even text descriptions of the syntheses and hardware set-up. To aid in the display and exploration, some derived information has been collected in a central location, such as yields and ratios. There is no guarantee that this derived information is complete, but the source information contains all recorded information if needed.
A second stumbling block has been a loss of implicit information in between the automated synthesis and the construction of the synthesis file. To clarify: when setting up the synthesis in the automated system, a lot of information comes from the researcher, is encoded in hardware, software, and tables of experimental conditions, to be subsequently translated into operating code. During synthesis, the automated platform then records a synthesis log in a plain log file (csv) as soon as anything happens. From this plain log file, the final synthesis file must be reconstructed. This reconstruction process has a lot of implicit information from the researcher in it, information that was “lost” between the configuration and the synthesis output file.
That means that we must spend some time to reconsider how to best administrate the configuration, operation and documentation of the syntheses. This should be a pragmatic, automated solution, ideally one that prevents too much loss of information. The word “ontologies” are thrown around copiously in these considerations, but as of yet have not proven to be an essential part of the solution just yet. So far, they ostensibly only add complications.
Without going too deep into the intricacies of the project however, please feel free to share our excitement and enjoyment at seeing the first batches of syntheses out there. Do download as many as you want and explore the structure using your favourite HDF5 structure exploration tool, and don’t hesitate to get in touch with Glen Smales and me.
These syntheses libraries will be extended soon with more batches, as well as their associated measurement libraries and analysis libraries, to make a rich dataset that can feed many data scientists in years to come.